NeurIPS competition tackles climate data challenges
The Earth’s climate is a highly complex, dynamic system. It is difficult to understand and predict how different climate variables interact. Finding causal relations in climate research today relies mostly on expensive and time-consuming model simulations. Fortunately, with the explosion in the availability of large-scale climate data and increasing computational power via the cloud, there are new, complementary ways to use machine learning (ML) and causal inference to understand relationships in climate data, like rainfall and ocean temperatures. This understanding can help improve weather forecasting and identify the causes of extreme events, like hurricanes and tornadoes. To help accelerate progress, AWS sponsored the Causality for Climate (C4C) competition at NeurIPS in 2019. This competition, which focused on the causal discovery and development of new methodologies to understand climate data, was one of 12 accepted NeurIPS 2019 competitions. It was organized by Jakob Runge and colleagues of the German Aerospace Center with collaborators at the University of Valencia.
Machine learning offers flexible methods that learn and adapt to the characteristics of climate data rather than assuming a rigid statistical model. This is important given the complex nature of climate data, which exhibits interdependencies between multiple subcomponents. Even with an unprecedented increase in the volume of climate observations, it is difficult to find patterns and identify complex relationships amongst the data. The goal of the competition was to develop new benchmarks and find new methods that can be applied to real-world challenges in climate. Participants were provided time series datasets featuring climate data (such as precipitation, humidity, and temperature) and AWS credits, with the aim to discover novel methodologies and open up new interdisciplinary research for climate data analysis.
The top prize went to a team of Ph.D.s and postdocs from the Copenhagen Causality Lab in the Department of Mathematical Sciences at the University of Copenhagen. They worked with 34 different datasets and the aim was to identify the causal relationship among the variables. The team started with simple baseline approaches and closely monitored the results as they introduced new variations to identify the methods that performed best across the competition track. Overall, because the climate data was blind and participants were not aware what measurements different time series corresponded to, they could optimize for the best methodologies without being influenced by preconceived hypotheses or assumptions. For more information, see their GitHub repo.
“Raising awareness in the community to focus on one of the most pressing issues of our time is a benefit of competitions like this,” said Sebastian Weichwald, a postdoc from the University of Copenhagen. “We are excited to see what was behind the competition data. As a next step, we would like to further investigate why the methods we employed performed well in this competition and continue to iterate and learn to ultimately make an impact on sustainability and climate science.”
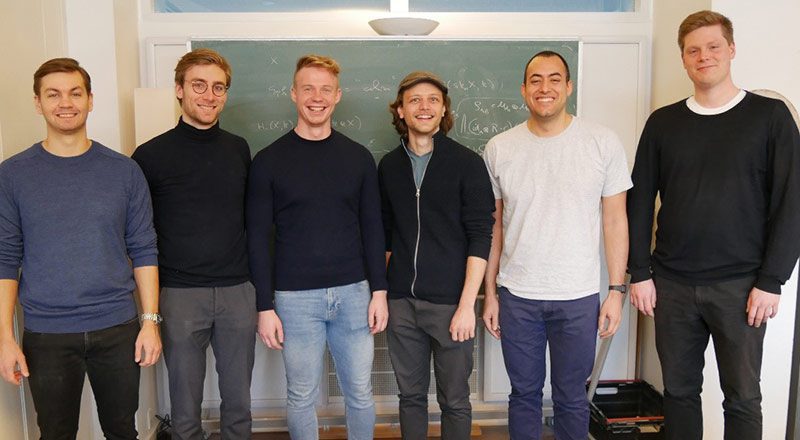
The winning team from University of Copenhagen. From left to right: Lasse Petersen, Nikolaj Thams, Phillip Bredahl Mogensen, Sebastian Weichwald, Gherardo Varando and Martin Emil Jakobsen.
A second winning team, made up of professors and Ph.D.s from the University of Ghent (Belgium), University of Palermo (Italy), University of Bari (Italy), and University of Rome La Sapienza (Italy), focused on the nonlinear nature of climate interactions. Their method was inspired by the theory of chaotic systems. This theory originated from studying our weather, which is a chaotic system in which you cannot forecast weather more than a few days out. The team used an approach that better captures those dynamics, which is why they succeeded in the categories with chaotic nonlinear datasets. Working to develop better tools to predict weather forecasting can help to understand climate change and extreme weather events. For more information, see their GitHub repo.
The winners were announced at NeurIPS on December 14, 2019. With 146 different methods and over 6,500 submitted results, the teams used AWS credits to iterate, experiment, and learn what methods delivered the best results. Their experimentation will help close the gap in understanding climate interactions and causality and raise awareness in a variety of communities, from physics to ML to statistics, to spur new innovation to improve upon our understanding of global climate.
About the author
Rebecca Wolff is a senior product marketing manager for AWS AI/ML. She is passionate about the power of machine learning to benefit society and improve lives. Out of the office, she likes to try new recipes and explore Seattle neighborhoods with her family.

Tags: Archive
Leave a Reply